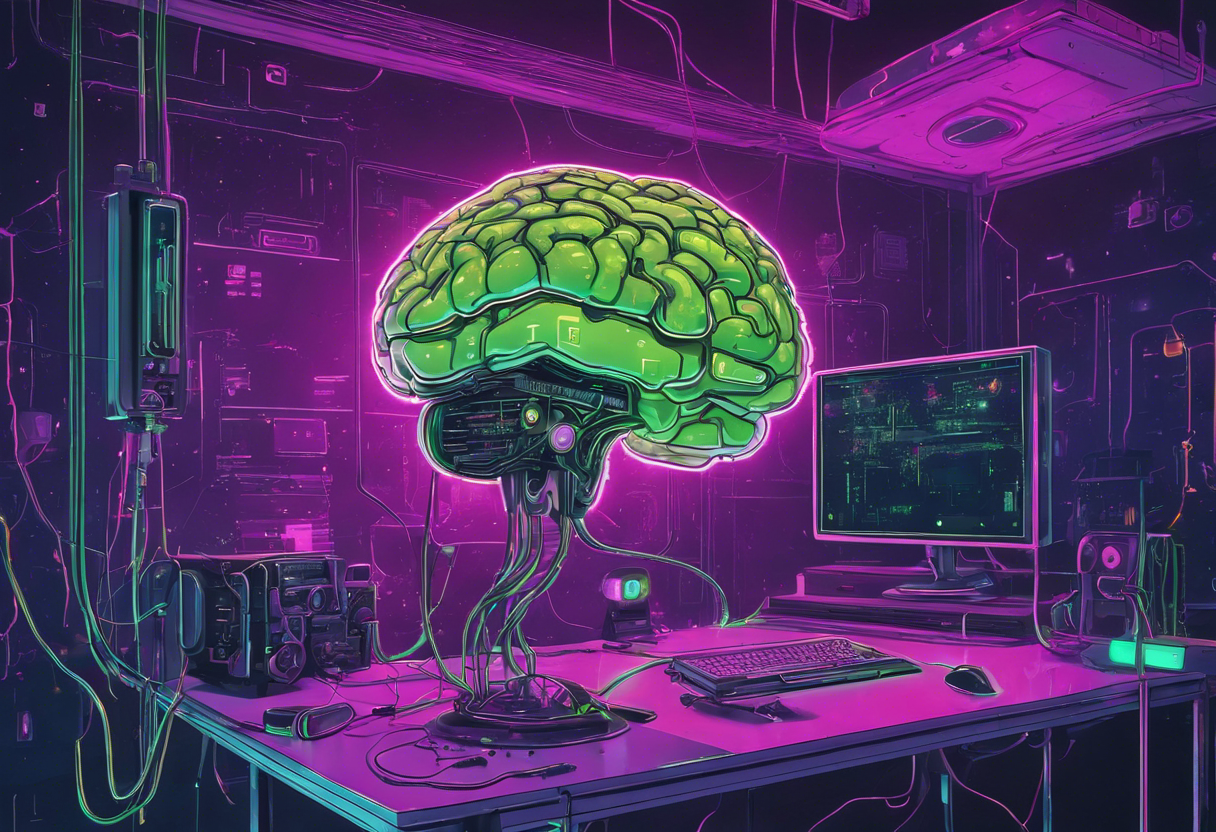
Navigating Seminal Papers and Tech Blocs in Deep Learning for AI Engineers
The ever-evolving landscape of Artificial Intelligence (AI) is a realm that beckons AI engineers towards groundbreaking innovation, constantly propelled by seminal papers and transformative technologies. In this dynamic sphere, where the limits of possibility are continually pushed, engineers delve into the depths of AI’s core concepts, unraveling revolutionary theories and groundbreaking applications.
Seminal papers such as “Attention Is All You Need” by Vaswani et al. have served as catalysts in the evolution of AI. This paper introduced the Transformer architecture, revolutionizing natural language processing (NLP) by employing the mechanism of attention. The concept of self-attention, a pivotal component of the Transformer model, enabled parallel processing of words in sentences, fundamentally reshaping how machines comprehend and generate human language.
Beyond the realm of attention mechanisms, the technological blocs within deep learning have reshaped the AI landscape:
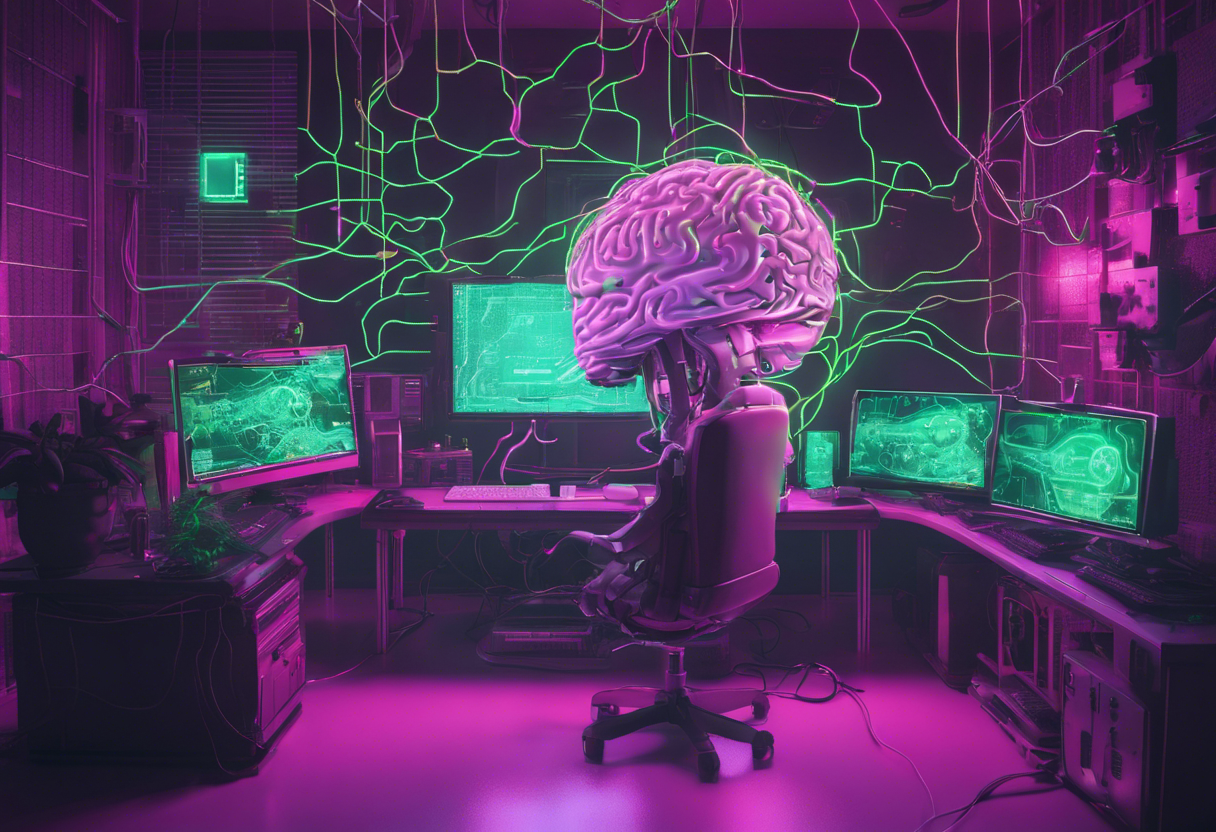
Our amazing team is always hard at work
While these technological blocs have significantly advanced AI capabilities, the journey of AI engineers goes beyond implementation. It entails deciphering complex architectures, refining model interpretability, and addressing ethical considerations deeply embedded within these advancements.
AI engineers are tasked not only with harnessing these powerful tools but also with grappling with challenges such as bias mitigation, model explainability, and ethical deployment. As they navigate these challenges, they actively engage in devising innovative solutions, striving to ensure that AI systems are not only powerful but also transparent, fair, and accountable.
Collaboration and interdisciplinary interactions form the bedrock of AI innovation. Engineers collaborate across domains, incorporating insights from psychology, ethics, sociology, and other fields, thereby enriching AI solutions with diverse perspectives and ethical considerations.
In this ongoing odyssey of AI innovation, engineers continually strive for deeper understandings, evolving methodologies, and ethical frameworks that transcend technological prowess. They are the vanguards, paving the path towards an AI future that not only astounds with its capabilities but also enriches and empowers humanity, ensuring that the strides taken in AI are symbiotic with societal values and ethical norms.